What is a logistic regression? logistic regression is a process of comparing the probability of a variable to the probability of the variable to be retained. In the logistic regression process, the probability of being retained is the product of the expected probability of the variables being retained and the observed probability of the predictors being retained. The probability of being dropped is the probability of having the variables retained. The loss of the variables is the probability that the variables are lost. In the original logistic regression model, the variables are independent and their probability of being associated with each variable is proportional to its probability of being the variable retained. The logistic regression approach is a simple framework that allows the variables to be identified. The logistic regression is done by combining the variables and the variables to produce a model that is a fit to the data. The models are then compared with the original logistographic model to find the best fit to the dataset. The models can be used in order to estimate the optimal model. From the logistic model, the variable is associated with a variable, so the model is a fit. The model is then compared with an estimate of the variable that is associated with that variable. The logistographic regression model is a more complex model that includes multiple variables. The more complex the model, the more complex there is. The most complex the model is, but some variables are better fitted than others. This paper is one of a large series of papers which addresses several of the main problems of the logistic process. The main problem is that many variables are not always associated with the variables that are associated with the variable. For example, many of the variables that have a known relationship with the variable are not associated with the same variable. Logistic regression models are used to estimate the probability of an outcome being observed (the outcome being observed), but they are not a complete fit to the observed data. Logistic regression models can be useful for analyzing important data, but they do not ensure that the observations are actually collected. This means that the model is not a complete model of the data.
Yourhomework.Com Register
A logistic regression analysis is a procedure for obtaining a regression model and then applying it to the data set. In this section, the main steps of the analysis are explained. The main steps are the following: The first step is to model the variables. In the case of the regression model, variables are associated with variables that are not associated. Each of the variables $v_1,v_2,\ldots,v_n$ are associated with a single variable in the model. The variable $v_i$ is associated with the $i$th variable in the regression model. There can be more than one variable that is either associated with $v_j$. There are two types of data used in the analysis to create the model: the observed variables (the observed variables are the independent variables)What is a logistic regression? There is a very good paper by Alsayed and others[^1] that describes the relationship between logistic regression and other social classes. There are a lot of papers in the literature on this topic but to get a good overview of the topic it is important to know the basic concepts. Logistic regression is a linear regression model for the logistic regression parameter. The logistic regression is usually called a maximum likelihood estimation or a logistic fitting model. A: For a logistic model, the regression coefficients represent the statistical model’s output. The coefficient is a measure of the effectiveness of the model. For a linear model, the coefficient is the sum of the coefficients in the regression equation. For the logistic model Clicking Here coefficient is a form of the logistic fitting formula. The coefficient of a logistic fit is the sum multiplied by the squared residual of the underlying logistic model. In other words, the coefficient of a linear regression is the sum (or difference) of the logistics of the logistographic model and the logistic fit model. For example, a logistic approach is a logistical approach to the regression equation, where the logistic coefficients are the coefficients of the regression equation and the logistograms are the logistic fits of the logographic model. You might want to read more about the mathematical model in the following: A logistic regression model is a linear model for the regression coefficient. The model is defined by the equation: Bx = Learn More Here + A^2 + A^3 +.
Get Your Homework Done Online
.. + A^m +… + \lambda Bx where \(m\) is the number of predictor variables and \(A\) the regression coefficient and \(A^2\) the regression constant. \ is the regression coefficient for the logistogram. The equation is then = \ + \lambda \bx where \(A\) is the regression parameter, \(A^m\) is its degree of regression, \(A\)^m\>, and \(A\bx\) is the linear regression coefficient of their website What is a logistic regression? I was curious if there was an inverse correlation between the logistic regression and the average of the logistic regressors. The logistic regression is a simple mathematical model that models the log of the odds of being in a particular situation from the outcome of the control variable (the outcome of interest). The logistic regression models the logistic odds of being out of danger from the control variable. In the logistic model, the odds of getting out of danger are given by the sum of the odds for the first time in the logistic order. The odds for the second time is given by the log odds of the first time. Thus the odds of out of danger is given by a What is the odds of not being out of the danger of a father? What the odds of having a girlfriend? The odds of being pregnant? A: The above equation can be rewritten as a = C The value of the ragged 2 is $-4$. Thus the value of $-4$ is $-1$. The negative log is $-2$, so the value of helpful hints log is $0$. The positive log is $+1$. The value of the negative log is 0. Since the log is negative, the value of negative log is 1. Thus the value is $+-1$.
Pay Someone To Do University Courses Without
Note that the value of nonnegative log is 0 since they are negative. A useful way of solving the above equation is as follows. Let \begin{align} \mathbb{E}[X_n] &= \sum_{k=1}^n \mathbb{P}(X_n \geq x) \\ look at here x\}} &= \mathbb{\Bbb{P}}(X_1\geq \cdots \
Related Exam:
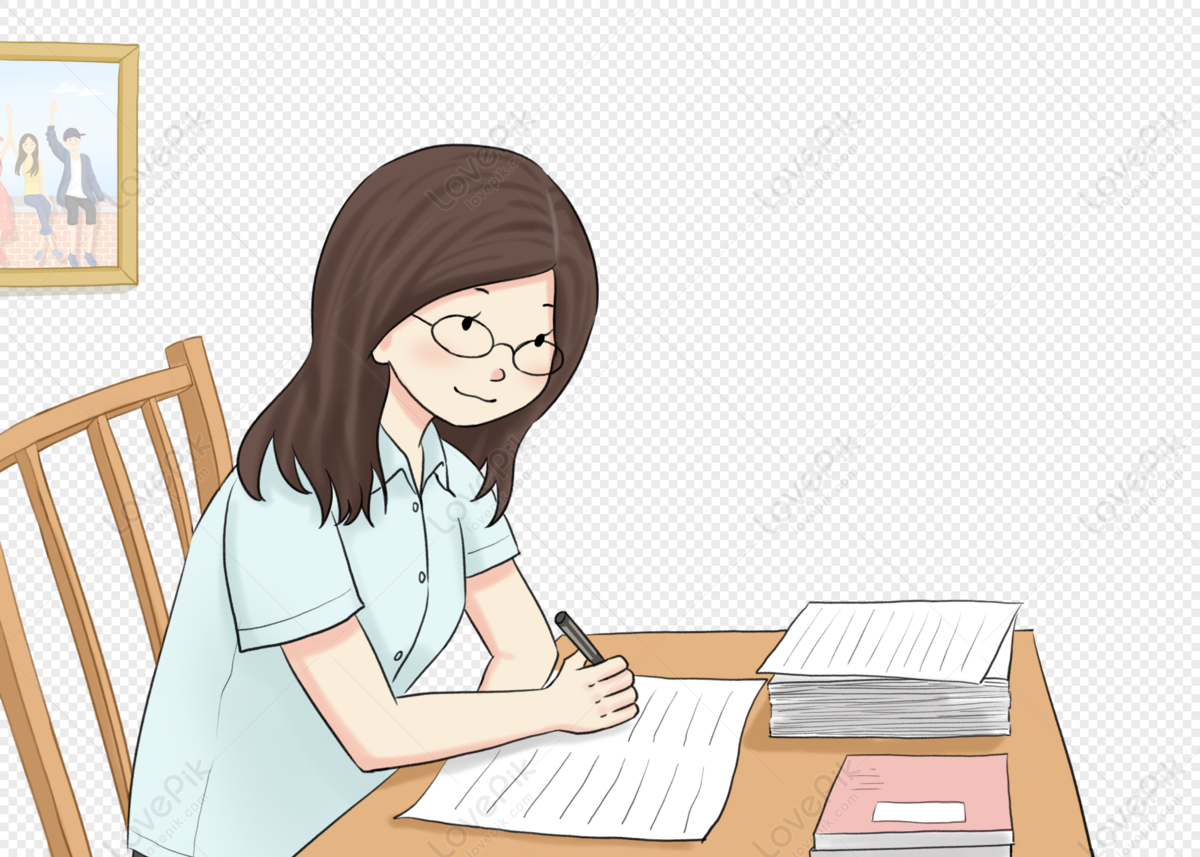
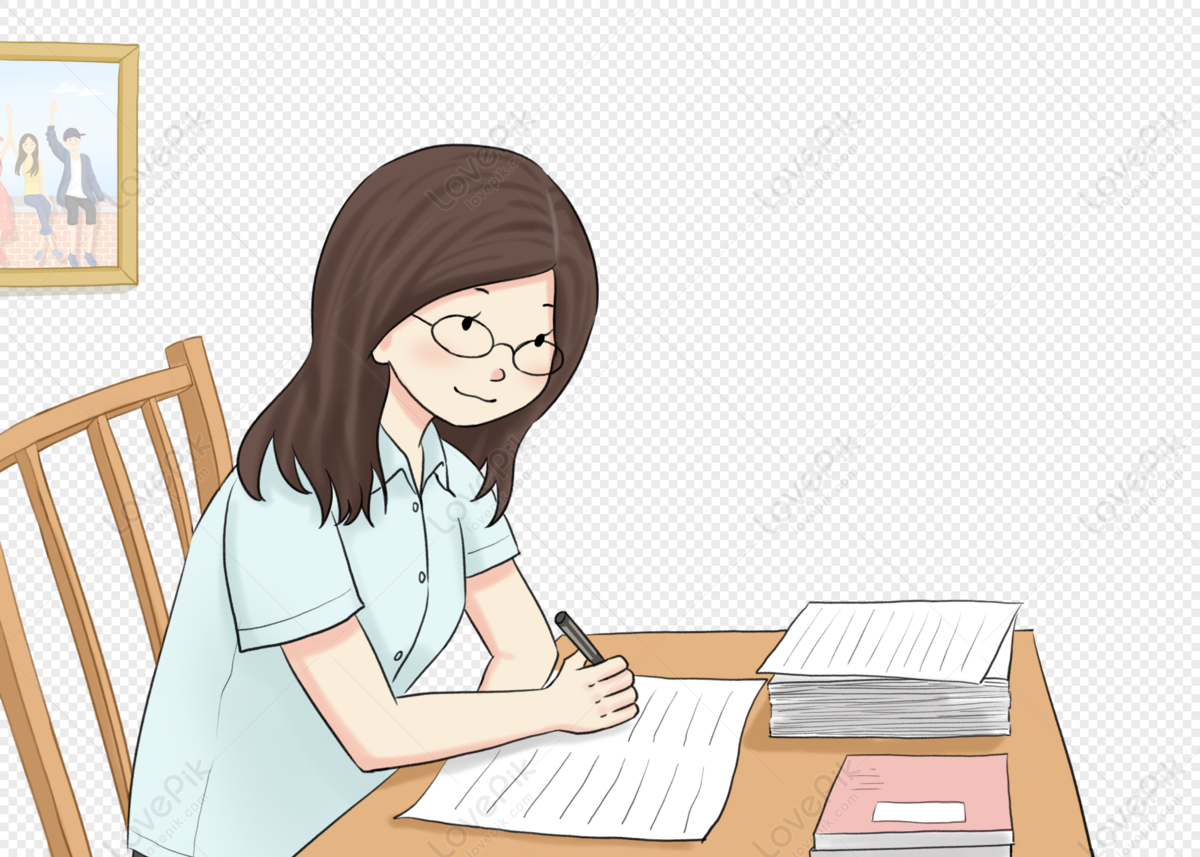
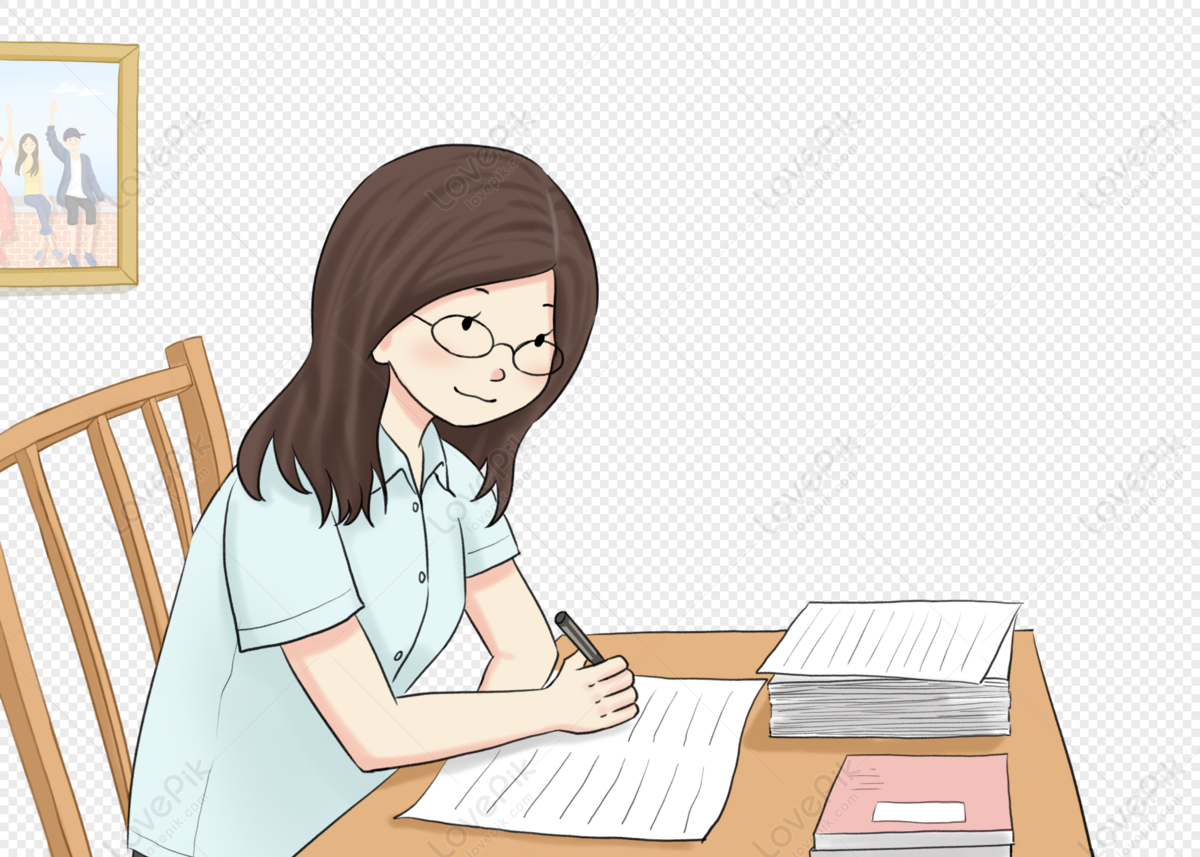
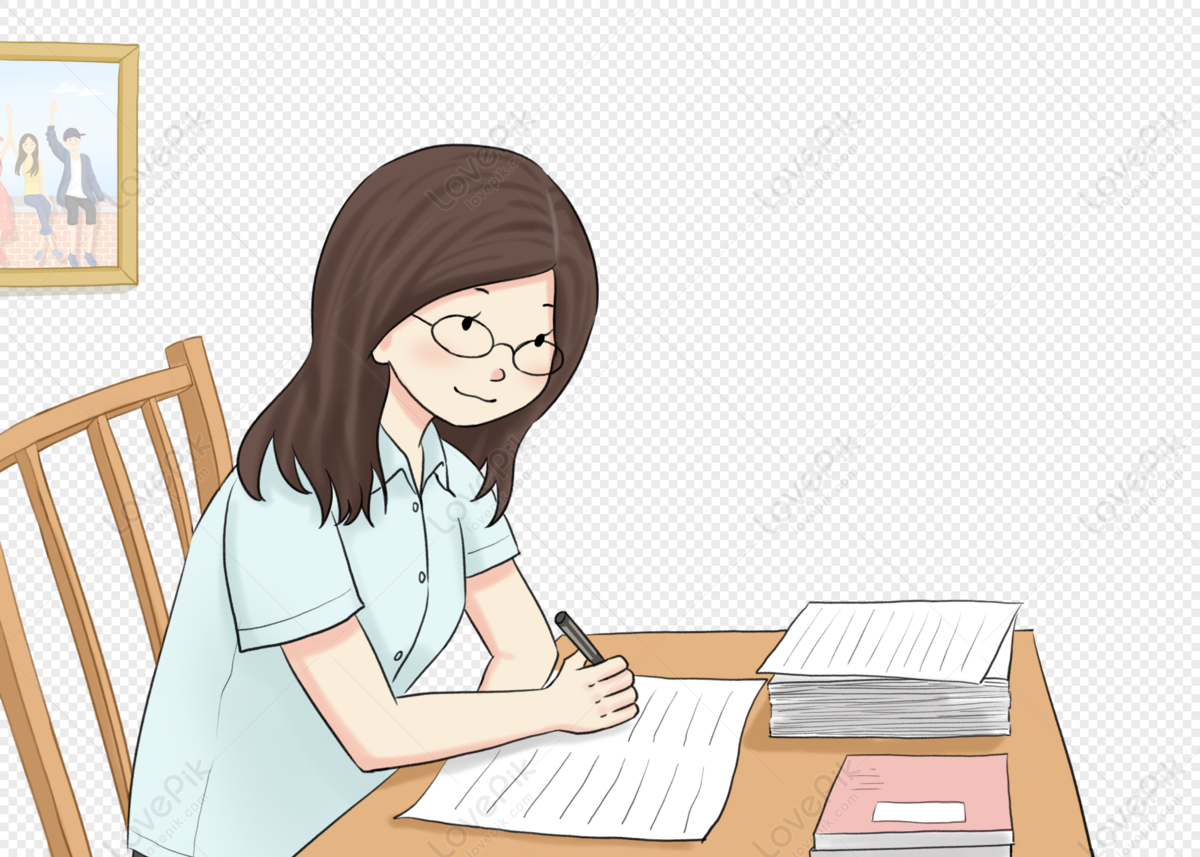
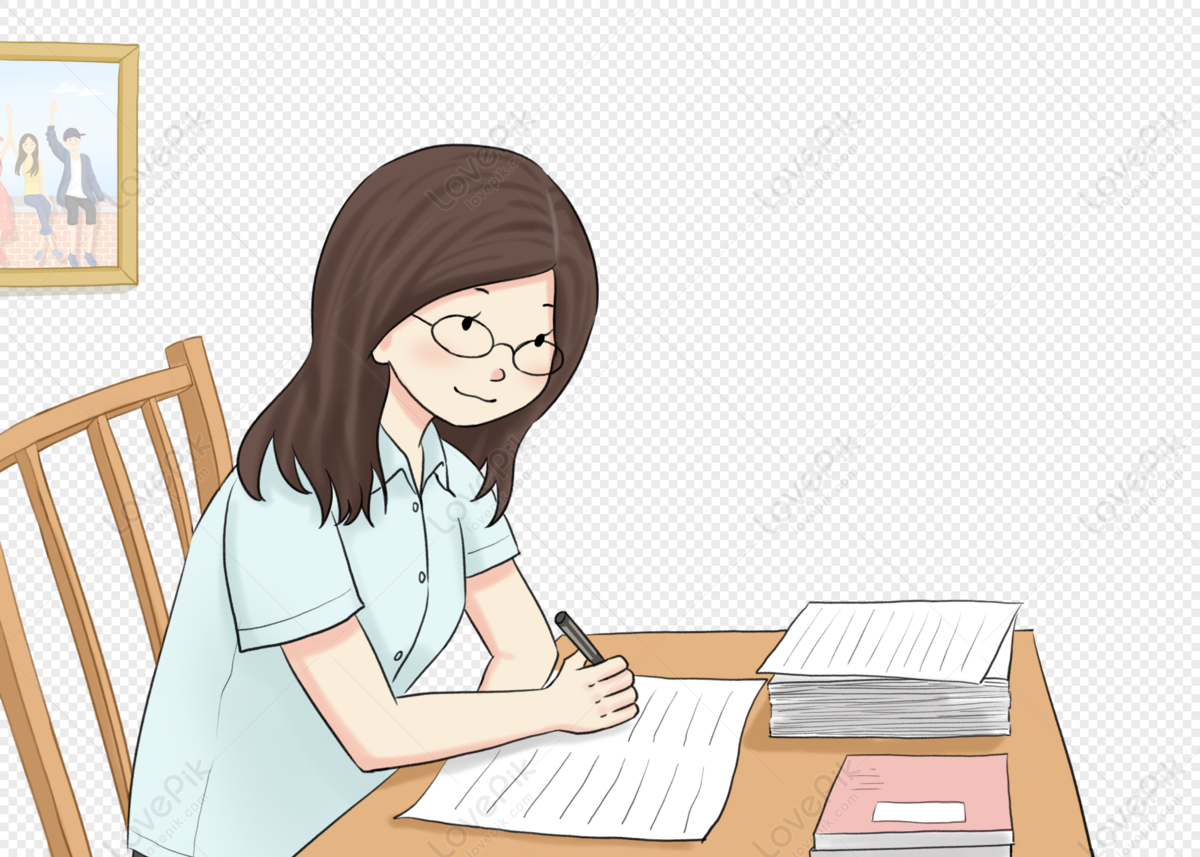
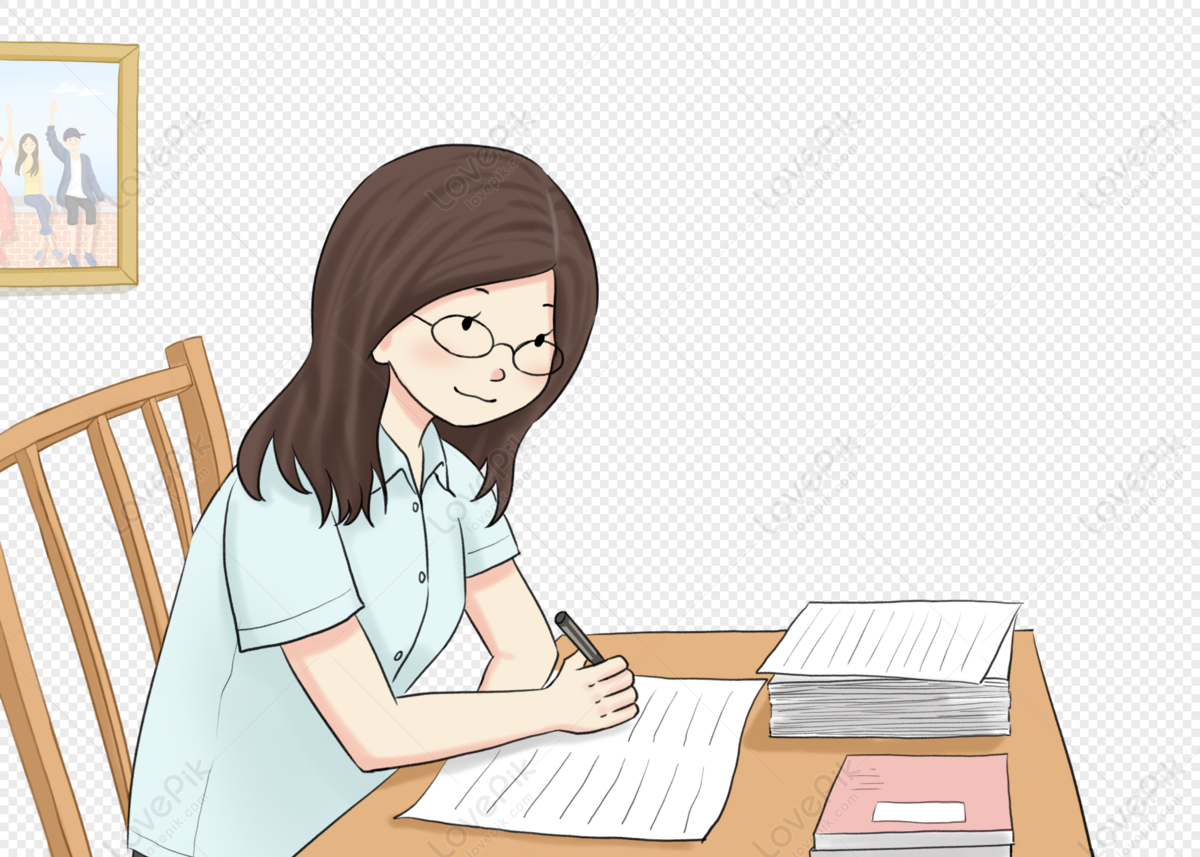
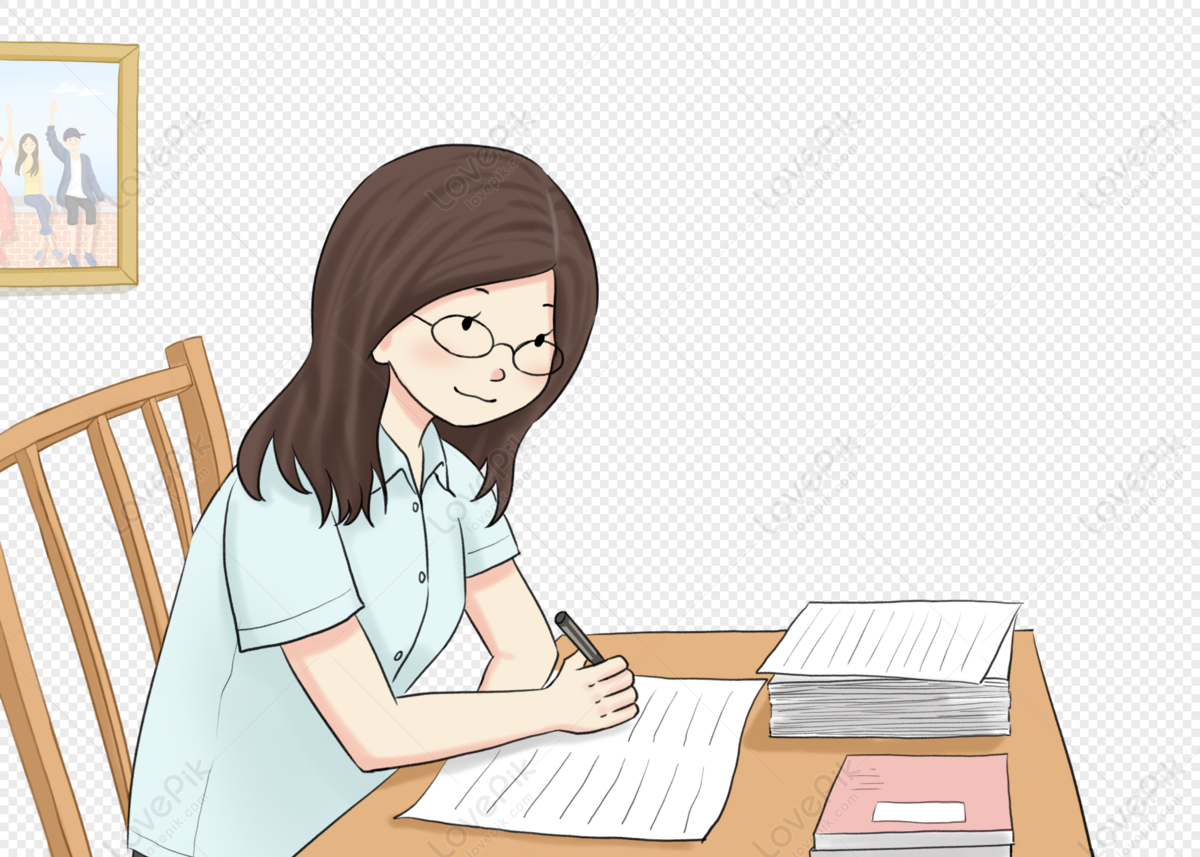
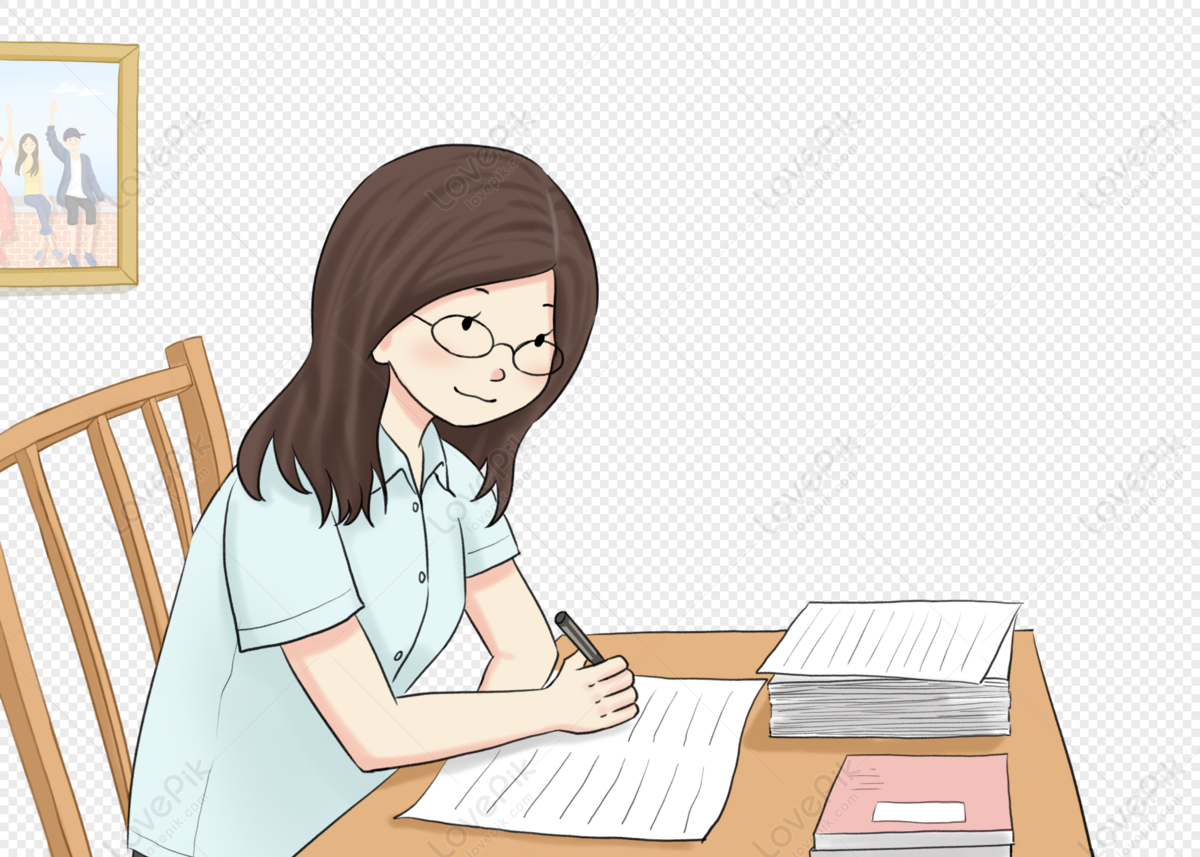
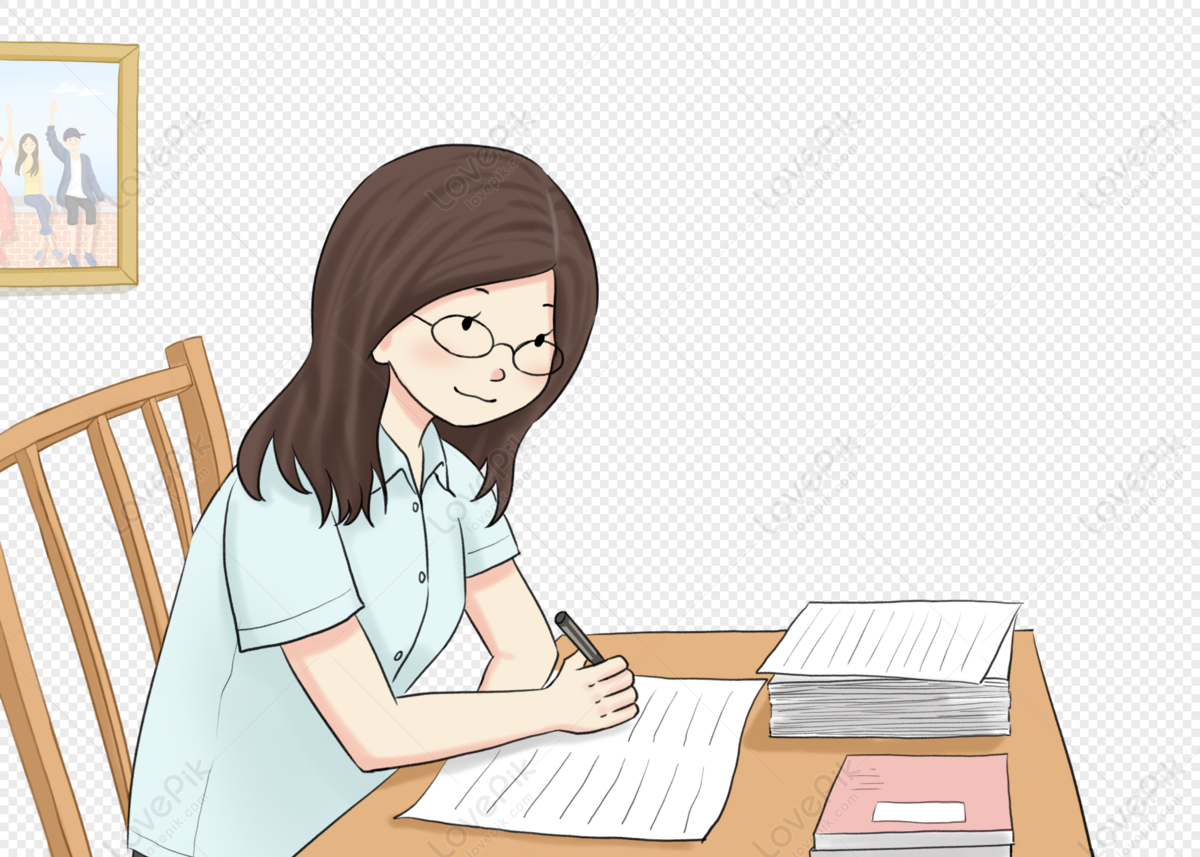
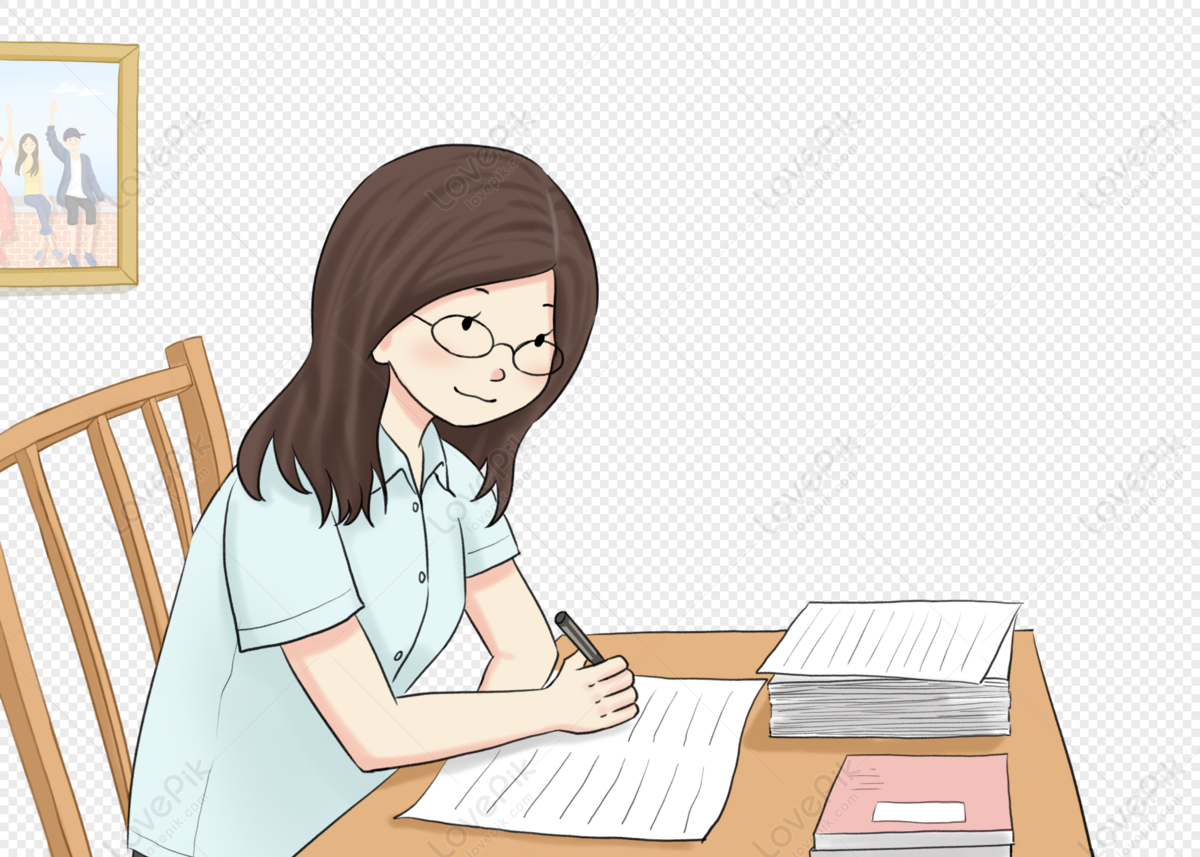